Plenary 1 | Wednesday 10 April 2024, 9:00-10:00
Learning in Machines
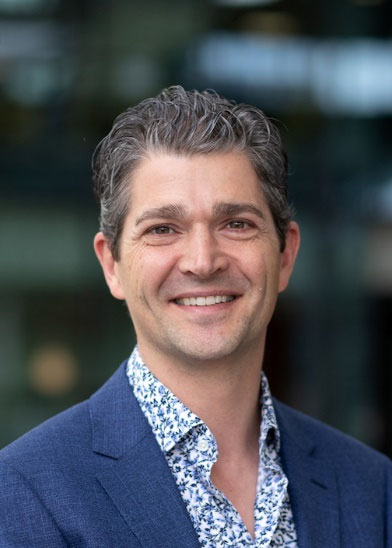
Professor Tom Oomen
Department of Mechanical Engineering, Eindhoven University of Technology
Faculty of Mechanical Engineering, Delft University of Technology
The Netherlands
Abstract
The future of manufacturing equipment and scientific instruments hinges on the ability to perform precise and fast motions. Examples of such mechatronic systems include wafer scanners, printing systems, pick-and-place machines, microscopes, and telescopes. These systems are subject to ever-increasing speed, accuracy, and flexibility requirements. Learning from data provides major opportunities to meet these requirements. In this presentation, I will outline new identification, learning, and control methodologies that can deal with the increasing requirements and large complexity in envisaged future mechatronic systems. I will also show their successful implementation on a selection of state-of-the-art mechatronic systems. The new results pave the way for new, revolutionary, data-intensive mechatronic designs with a massive number of actuators and sensors.
Biography
Tom Oomen is full professor with the Department of Mechanical Engineering at the Eindhoven University of Technology. He is also a part-time full professor with the Delft University of Technology. He received the M.Sc. degree (cum laude) and Ph.D. degree from the Eindhoven University of Technology, Eindhoven, The Netherlands. He held visiting positions at KTH, Stockholm, Sweden, and at The University of Newcastle, Australia. He is a recipient of the 7th Grand Nagamori Award, the Corus Young Talent Graduation Award, the IFAC 2019 TC 4.2 Mechatronics Young Research Award, the 2015 IEEE Transactions on Control Systems Technology Outstanding Paper Award, the 2017 IFAC Mechatronics Best Paper Award, the 2019 IEEJ Journal of Industry Applications Best Paper Award, and recipient of a Veni and Vidi personal grant. He is currently a Senior Editor of IEEE Control Systems Letters (L-CSS) and Co-Editor-in-Chief of IFAC Mechatronics, and he has served on the editorial board of IEEE Transactions on Control Systems Technology. He is also vice-chair for IFAC TC 4.2. His research interests are in the field of data-driven modeling, learning, and control, with applications in precision mechatronics.
Plenary 2 | Thursday 11 April 2024, 9:00-10:00
Dynamical Systems Analysis using Sparse Polynomial Optimisation
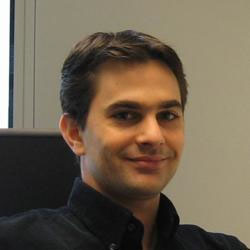
Professor Antonis Papachristodoulou
Department of Engineering Science
University of Oxford
The United Kingdom
Abstract
Many problems in robust and nonlinear control can be formulated using polynomial positivity conditions: the simplest example is the search of polynomial Lyapunov functions for stability analysis of equilibria of dynamical systems with polynomial vector fields. The discovery that semidefinite programming can be used to test polynomial non-negativity, using sum of squares, opens up new directions in nonlinear systems analysis and design. In this talk I will first present how ideas from dynamical systems, positive polynomials and convex optimization can be used to analyse the stability, robust stability, performance and robust performance of systems described by nonlinear ODEs.
Although entirely algorithmic, this approach does not scale well to large system instances, but Chordal Sparsity methods offer a way to address this challenge. I will motivate and use this decomposition method on the problem of understanding the properties of Neural Networks and Neural Feedback Loops. Indeed, it is becoming clear that providing robust guarantees on systems that use neural networks is very important, especially in safety-critical applications given that a trained neural network's sensitivity to adversarial attacks is one of its greatest shortcomings. To provide robust guarantees, one popular method is to describe and bound the activation functions in the neural network using equality and inequality constraints. However, there are numerous ways to form these bounds, providing a trade-off between conservativeness and complexity. I will first show that Sum of Squares methods can tightened bounds significantly, whilst the computational time remains reasonable, using Chordal Sparsity methods and then consider the problem of analysing neural feedback loops using structured Lyapunov functions and sum of squares, demonstrating that this method can improve estimates on the region of attraction of an equilibrium.
Biography
Antonis joined the University of Oxford in 2006, where he is currently Professor of Engineering Science and a Tutorial Fellow in Worcester College, Oxford. He was previously EPSRC Fellow and Director of the EPSRC & BBSRC Centre for Doctoral training in Synthetic Biology. He obtained an MA/MEng degree in Electrical and Information Sciences from the University of Cambridge and a PhD in Control and Dynamical Systems from the California Institute of Technology, with a PhD Minor in Aeronautics. In 2015 he was awarded the European Control Award for his contributions to robustness analysis and applications to networked control systems and systems biology and the O. Hugo Schuck Best Paper Award. He is IEEE Fellow for his contributions to the analysis and design of networked control systems. He serves regularly on Technical Programme Committees for conferences and was associate editor for Automatica and IEEE Transactions on Automatic Control.
Plenary 3 | Friday 12 April 2024, 9:00-10:00
An Automotive Odyssey: Algorithms, Mechanisms and Estimation
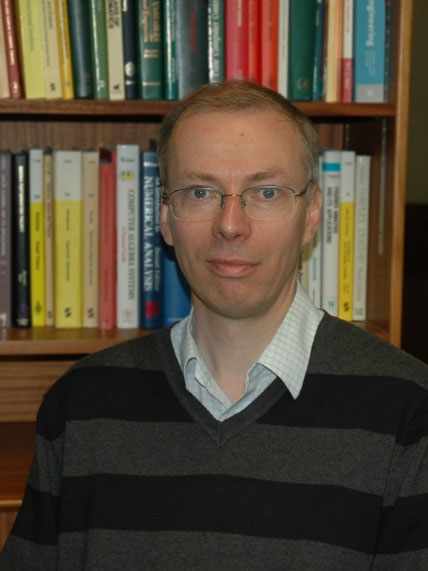
Professor Malcolm Smith
Department of Engineering
University of Cambridge
The United Kingdom
Abstract
The talk begins with a discussion of the approach, theory and stochastic optimal control algorithm that was developed for the McLaren 720S semi-active suspension control system together with Panos Brezas and Will Hoult. The second part of the talk develops further the ideas of adjustable mechanical devices including the principles and realisability of losslessly adjustable one-ports with reference to recent work with Tryphon Georgiou and Faryar Jabbari. The final part of the talk connects with the first theme and describes recent work together with Greg Gakis on simultaneous input and state estimation.
Biography
Malcolm Smith received the B.A. (M.A.) degree in mathematics, the M.Phil in control engineering and operational research and the Ph.D. degree in control engineering from the University of Cambridge, England. He was subsequently a Research Fellow at the German Aerospace Centre, DLR, Oberpfaffenhofen, Germany, a Visiting Assistant Professor and Research Fellow with the Department of Electrical Engineering at McGill University, Montreal, Canada, and an Assistant Professor with the Department of Electrical Engineering at the Ohio State University, Columbus, USA. He is now a Fellow of Gonville and Caius College and a Professor in the Department of Engineering at the University of Cambridge. He received the 1992 and 1999 George Axelby Best Paper Awards, in the IEEE Transactions on Automatic Control in 1992 and 1999, both times for joint work with T.T. Georgiou. He was awarded the Sir Harold Hartley Medal 2009 (Institute of Measurement and Control) for "outstanding contribution to the technology of measurement and control". He is a Fellow of the IEEE and a Fellow of the Royal Academy of Engineering.